이 기사는 대규모 언어 모델(LLM)을 사용하여 데이터가 많지 않은 경우 NER 모델을 학습하는 방법에 대해 논의합니다.
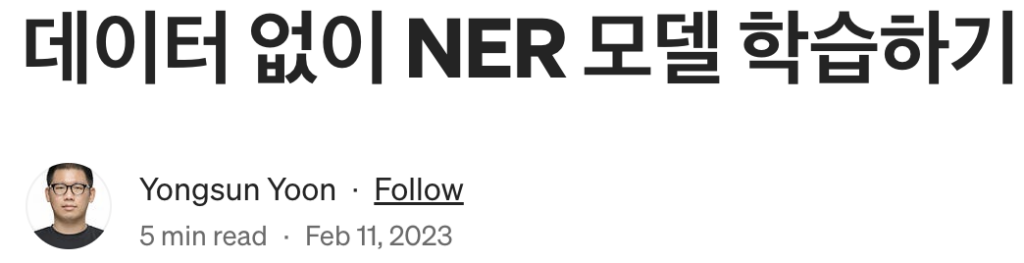
몇 가지 예제 엔티티 목록을 사용하여 NER 데이터 세트를 생성하는 프로세스를 설명합니다. 이 프로세스에는 1) Few Shot 엔티티 목록을 만드는 것, 2) GPT-3를 사용하여 이를 확장하는 것, 3) GPT-3를 사용하여 NER 데이터 세트를 생성하는 것, 4) 그리고 이 생성된 데이터로 BERT 모델을 학습하는 것이 포함됩니다.
저자는 호텔 도메인을 예로 사용하여 5개의 엔티티 클래스를 정의하고, 클래스당 100개의 예제를 생성했습니다. 결과는 검증 데이터에서 유망한 성능을 보여주었습니다.
이 방법에서 두 가지만 변경하여 실행한 학습 코드와 추가로 분류 코드를 제공하고자 합니다. LLM을 활용하여 데이터셋을 자동 확장한 좋은 방법이라 생각됩니다.
1) GPT대신 한국어 무료 모델 Exaone3.5 모델 적용.
2) GPU 대신 CPU 실행
학습 코드
import re
import ollama
import numpy as np
import torch
import itertools
import torch.nn.functional as F
from tqdm import tqdm
from seqeval.scheme import IOB2
from transformers import AutoTokenizer, AutoModelForTokenClassification
from sklearn.metrics import f1_score
from seqeval.metrics import f1_score as ner_f1_score
real_entities = [
{
'class_name': '인명',
'entity_names': [
'홍길동',
'이순신',
'강감찬',
'이승만',
'이율곡'
]
},
{
'class_name': '지명',
'entity_names': [
'대한민국',
'서울특별시',
'강남구',
'삼성동',
'음성읍',
]
},
{
'class_name': '시간',
'entity_names': [
'2025년',
'3월',
'25일',
'10시',
'2025년 3월 25일 10시 25분 30초'
]
},
{
'class_name': '기업',
'entity_names': [
'삼성전자',
'SK하이닉스',
'포스코',
'이마트',
'GS리테일'
]
},
{
'class_name': '질병',
'entity_names': [
'식도역류증',
'위염',
'과민성 대장 증후군',
'감기',
'당뇨병'
]
}
]
def generate(prompt, model='exaone3.5', max_tokens=512):
# Ollama's generate method
response = ollama.generate(
model=model,
prompt=prompt,
options={"max_tokens": max_tokens}
)
# Extract the response text
return response['response']
def construct_entity_prompt(class_name, entity_names, k=10):
prompt = f'<{class_name}> 개의 엔터티 이름이 있습니다. {k}개의 새로운 <{class_name}> 엔터티 이름을 생성합니다.\n\n'
prompt += 'Entity names:\n'
for e in entity_names:
prompt += f'- {e}\n'
prompt += '\nGenerated names:\n-'
return prompt
def postprocess_entities(synthetic_entities):
processed = []
for ents in synthetic_entities:
ents = ents.split('\n')
for e in ents:
if '- ' in e: # Process only valid entries
processed.append(e.split('- ')[1].strip())
return processed
synthetic_entities = []
for real_ent in tqdm(real_entities):
class_name, entity_names = real_ent['class_name'], real_ent['entity_names']
prompt = construct_entity_prompt(class_name, entity_names)
syn_entities = generate(prompt)
syn_entities = postprocess_entities([syn_entities])
syn_entities = list(set(syn_entities)) # Remove duplicates
synthetic_entities.append({'class_name': class_name, 'entity_names': syn_entities})
all_entities = []
for real, synthetic in zip(real_entities, synthetic_entities):
all_entities.append({
'class_name': real['class_name'],
'entity_names': list(set(real['entity_names'] + synthetic['entity_names']))
})
def sample_entities(all_entities, min_k=1, max_k=3):
k = np.random.randint(min_k, max_k + 1)
idxs = np.random.choice(range(len(all_entities)), size=k, replace=False)
entities = []
for i in idxs:
ents = all_entities[i]
name = np.random.choice(ents['entity_names'])
entities.append({'class_name': ents['class_name'], 'entity_name': name})
return entities
def construct_sentence_prompt(entities, style='dialog'):
prompt = f'엔터티를 포함하는 {style} 문장을 생성합니다.\n\n'
entities_string = ', '.join([f"{e['entity_name']}({e['class_name']})" for e in entities])
prompt += f'엔터티: {entities_string}\n'
prompt += 'Sentence:'
return prompt
def construct_labels(generated, entities, class2idx):
labels = [class2idx['outside']] * len(generated)
for ent in entities:
l = class2idx[ent['class_name']]
for span in re.finditer(ent['entity_name'].lower(), generated.lower()):
s, e = span.start(), span.end()
labels[s] = l
labels[s + 1:e] = [l + 1] * (e - s - 1)
return labels
class2idx = {e['class_name']: i * 2 for i, e in enumerate(all_entities)}
class2idx['outside'] = len(class2idx) * 2
data = []
for _ in tqdm(range(100)):
batch_data = []
for _ in range(10):
batch_entities = sample_entities(all_entities)
batch_prompt = construct_sentence_prompt(batch_entities)
generated_text = generate(batch_prompt)
labels = construct_labels(generated_text, batch_entities, class2idx)
batch_data.append({'text': generated_text, 'labels': labels})
data.extend(batch_data)
print(data)
LABELS = ['B-PERSON', 'I-PERSON', 'B-REGION', 'I-REGION', 'B-DATETIME', 'I-DATETIME', 'B-CORPORATION', 'I-CORPORATION', 'B-DISEASE', 'I-DISEASE', 'O']
def pad_sequences(seqs, pad_val, max_length):
_max_length = max([len(s) for s in seqs])
max_length = min(max_length, _max_length)
padded_seqs = []
for seq in seqs:
seq = seq[:max_length]
pads = [pad_val] * (max_length - len(seq))
seq = seq + pads
padded_seqs.append(seq)
return padded_seqs
class Dataset(torch.utils.data.Dataset):
def __init__(self, data, tokenizer, max_length, split='train'):
self.data = data
self.tokenizer = tokenizer
self.max_length = max_length
self.split = split
def __len__(self):
return len(self.data)
def __getitem__(self, idx):
item = self.data[idx]
text = item['text']
char_labels = item['labels']
inputs = self.tokenizer(text)
input_ids = inputs.input_ids
attention_mask = inputs.attention_mask
labels = []
for i in range(len(input_ids)):
span = inputs.token_to_chars(i)
if span is None:
labels.append(len(LABELS) - 1) # O
else:
labels.append(char_labels[span.start])
return input_ids, attention_mask, labels
def collate_fn(self, batch):
input_ids, attention_mask, labels = zip(*batch)
input_ids = pad_sequences(input_ids, self.tokenizer.pad_token_id, self.max_length)
attention_mask = pad_sequences(attention_mask, 0, self.max_length)
labels = pad_sequences(labels, -100, self.max_length)
return torch.tensor(input_ids), torch.tensor(attention_mask), torch.tensor(labels)
tokenizer = AutoTokenizer.from_pretrained('roberta-base', clean_up_tokenization_spaces=True)
rand_idxs = np.random.permutation(range(len(data)))
train_idxs = rand_idxs[100:]
valid_idxs = rand_idxs[:100]
train_data = [data[i] for i in train_idxs]
valid_data = [data[i] for i in valid_idxs]
train_dataset = Dataset(train_data, tokenizer, 256)
train_loader = torch.utils.data.DataLoader(train_dataset, batch_size=16, shuffle=True, collate_fn=train_dataset.collate_fn)
valid_dataset = Dataset(valid_data, tokenizer, 256)
valid_loader = torch.utils.data.DataLoader(valid_dataset, batch_size=16, shuffle=False, collate_fn=valid_dataset.collate_fn)
def train(model, loader, device, outside_weight=0.9):
model.train()
label_weight = torch.ones(model.num_labels)
label_weight[-1] = outside_weight
label_weight = label_weight.to(device)
pbar = tqdm(loader)
for batch in pbar:
batch = [b.to(device) for b in batch]
input_ids, attention_mask, labels = batch
outputs = model(input_ids, attention_mask)
logits = outputs.logits
logits = logits.view(-1, model.num_labels)
labels = labels.view(-1)
loss = F.cross_entropy(logits, labels, weight=label_weight)
optimizer.zero_grad()
loss.backward()
optimizer.step()
pbar.set_postfix({'loss': loss.item()})
def predict(model, loader, device):
model.eval()
total_preds, total_labels = [], []
for batch in tqdm(loader):
batch = [b.to(device) for b in batch]
input_ids, attention_mask, labels = batch
with torch.no_grad():
outputs = model(input_ids, attention_mask, labels=labels)
preds = outputs.logits.argmax(dim=-1)
total_preds += preds.cpu().tolist()
total_labels += labels.cpu().tolist()
return total_preds, total_labels
def remove_padding(preds, labels):
removed_preds, removed_labels = [], []
for p, l in zip(preds, labels):
if -100 not in l: continue
idx = l.index(-100)
removed_preds.append(p[:idx])
removed_labels.append(l[:idx])
return removed_preds, removed_labels
def entity_f1_func(preds, targets):
preds = [[LABELS[p] for p in pred] for pred in preds]
targets = [[LABELS[t] for t in target] for target in targets]
entity_macro_f1 = ner_f1_score(targets, preds, average="macro", mode="strict", scheme=IOB2)
f1 = entity_macro_f1 * 100.0
return round(f1, 2)
def char_f1_func(preds, targets):
label_indices = list(range(len(LABELS)))
preds = list(itertools.chain(*preds))
targets = list(itertools.chain(*targets))
f1 = f1_score(targets, preds, labels=label_indices, average='macro', zero_division=True) * 100.0
return round(f1, 2)
def evaluate(model, loader, device):
preds, labels = predict(model, loader, device)
preds, labels = remove_padding(preds, labels)
entity_f1 = entity_f1_func(preds, labels)
char_f1 = char_f1_func(preds, labels)
return entity_f1, char_f1
num_labels = len(LABELS)
id2label = {i: l for i, l in enumerate(LABELS)}
label2id = {l: i for i, l in enumerate(LABELS)}
model = AutoModelForTokenClassification.from_pretrained('roberta-base', num_labels=num_labels, id2label=id2label, label2id=label2id)
_ = model.train().to('cpu')
optimizer = torch.optim.Adam(model.parameters(), lr=5e-5)
best_score = 0.
for ep in range(5):
train(model, train_loader, 'cpu')
entity_f1, char_f1 = evaluate(model, valid_loader, 'cpu')
print(f'단계: {ep:02d} | 엔터티 f1: {entity_f1:.2f} | 단어 f1: {char_f1:.2f}')
if entity_f1 > best_score:
model.save_pretrained('checkpoint')
tokenizer.save_pretrained('checkpoint')
best_score = entity_f1
<학습 결과>
100%|██████████| 57/57 [03:29<00:00, 3.68s/it, loss=0.55]
100%|██████████| 7/7 [00:05<00:00, 1.28it/s]
단계: 00 | 엔터티 f1: 0.00 | 단어 f1: 15.53
100%|██████████| 57/57 [03:33<00:00, 3.75s/it, loss=0.142]
100%|██████████| 7/7 [00:06<00:00, 1.10it/s]
단계: 01 | 엔터티 f1: 23.61 | 단어 f1: 51.36
100%|██████████| 57/57 [03:36<00:00, 3.80s/it, loss=0.122]
100%|██████████| 7/7 [00:05<00:00, 1.24it/s]
단계: 02 | 엔터티 f1: 91.15 | 단어 f1: 92.38
100%|██████████| 57/57 [03:33<00:00, 3.74s/it, loss=0.0128]
100%|██████████| 7/7 [00:05<00:00, 1.28it/s]
단계: 03 | 엔터티 f1: 96.15 | 단어 f1: 97.31
100%|██████████| 57/57 [03:31<00:00, 3.71s/it, loss=0.00991]
100%|██████████| 7/7 [00:05<00:00, 1.28it/s]
단계: 04 | 엔터티 f1: 97.51 | 단어 f1: 97.90
분류 코드
import torch
from transformers import AutoTokenizer, AutoModelForTokenClassification
# 체크포인트 로드
checkpoint_path = 'checkpoint'
tokenizer = AutoTokenizer.from_pretrained(checkpoint_path)
model = AutoModelForTokenClassification.from_pretrained(checkpoint_path)
# 레이블 정의
LABELS = ['B-PERSON', 'I-PERSON', 'B-REGION', 'I-REGION', 'B-DATETIME',
'I-DATETIME', 'B-CORPORATION', 'I-CORPORATION',
'B-DISEASE', 'I-DISEASE', 'O']
def predict_ner(model, tokenizer, sentence):
# 토크나이징 (오프셋 정보 포함)
inputs = tokenizer(
sentence,
return_tensors="pt",
return_offsets_mapping=True,
return_special_tokens_mask=True,
add_special_tokens=True
)
# 워드 ID는 tokenizer의 word_ids 메소드로 별도 획득
word_ids = inputs.word_ids(0)
input_ids = inputs.input_ids
attention_mask = inputs.attention_mask
offsets = inputs.offset_mapping[0].numpy()
special_tokens_mask = inputs.special_tokens_mask[0].numpy()
# 예측 수행
with torch.no_grad():
outputs = model(input_ids, attention_mask=attention_mask)
predictions = torch.argmax(outputs.logits, dim=-1)[0].numpy()
# 엔티티 추출 및 병합
return extract_entities(sentence, offsets, predictions, word_ids, special_tokens_mask)
def extract_entities(sentence, offsets, predictions, word_ids, special_tokens_mask):
"""
원본 텍스트에서 엔티티 추출 및 같은 단어에 속한 토큰 병합
"""
entities = []
current_entity = None
prev_word_id = None
# 단어 ID별로 토큰 위치 및 예측 정보 수집
word_info = {}
for i, (offset, pred, word_id, is_special) in enumerate(
zip(offsets, predictions, word_ids, special_tokens_mask)):
# 특수 토큰 건너뛰기
if is_special:
continue
start, end = offset
label = LABELS[pred]
# 단어 ID별 정보 수집
if word_id not in word_info:
word_info[word_id] = {
'start': start,
'end': end,
'labels': [label]
}
else:
word_info[word_id]['end'] = end
word_info[word_id]['labels'].append(label)
# 단어별로 최종 레이블 결정
word_entities = []
for word_id, info in word_info.items():
start = info['start']
end = info['end']
text = sentence[start:end]
# 우선순위: B- > I- > O
label = 'O'
for l in info['labels']:
if l.startswith('B-'):
label = l
break
elif l.startswith('I-') and label == 'O':
label = l
if label != 'O':
entity_type = label[2:] # "B-" 또는 "I-" 제거
word_entities.append((word_id, entity_type, text, start, end))
# 연속된 같은 타입의 엔티티 병합
current_entity = None
merged_entities = []
for i, (word_id, entity_type, text, start, end) in enumerate(sorted(word_entities, key=lambda x: x[0])):
if current_entity is None:
current_entity = (entity_type, text, start, end)
elif current_entity[0] == entity_type and start <= current_entity[3] + 1:
# 같은 타입이고 가까운 위치에 있으면 병합
merged_text = sentence[current_entity[2]:end]
current_entity = (entity_type, merged_text, current_entity[2], end)
else:
merged_entities.append(current_entity)
current_entity = (entity_type, text, start, end)
if current_entity:
merged_entities.append(current_entity)
# 결과 형식 정리 (중복 제거)
result_dict = {}
for entity_type, text, start, end in merged_entities:
key = f"{start}_{end}_{entity_type}"
if key not in result_dict:
result_dict[key] = (entity_type, text)
return list(result_dict.values())
# 테스트 문장
sentence = "이순신 장군은 서울특별시에서 감기에 걸렸습니다."
entities = predict_ner(model, tokenizer, sentence)
# 결과 출력
print(f"Sentence: {sentence}")
print("Entities:")
for entity_type, entity_name in entities:
print(f" - {entity_name} ({entity_type})")
<분류 결과>
Sentence: 이순신 장군은 서울특별시에서 감기에 걸렸습니다.
Entities:
- 이순신 (PERSON)
- 서울특별시에서 (REGION)
- 감기에 (DISEASE)
Sentence: 1592년의 경상남도 거제현에서의 옥포해전은 이순신의 첫 승전을 알리게 된 해전이다.
Entities:
- 1592년의 (DATETIME)
- 이순신의 (PERSON)